Our team has reviewed thousands of early-stage AI startups over the past year, and we see a concerning pattern emerging — one that could result in countless startup failures in the near future.
This is mainly due to a lack of differentiation or competitive advantage (i.e., no “moat”) in these products. There are still far too many startups simply adding thin UI layers or light analytics atop an existing LLM like ChatGPT; this won’t be enough to compete with the Goliaths of the tech world or even other startups, many of whom have enough funding, data, and resources to create their own generic or fine-tuned AI models.
The good news is that we have created a framework beneficial for early-stage AI startups looking to build something much more differentiated and VC-fundable. While AI will create a lot of opportunities across the full tech stack in many sectors, this playbook focuses specifically on the subset of software companies looking to build and sell applied AI (e.g., machine learning capabilities) to a corporate client in order to solve a specific problem that they are facing.
In this segment of AI products, we are convinced that targeting the right early-adopter customer profiles will be critical, and that they will mostly depend on advantageous data partnerships. And, though they may be considered a foregone conclusion, the winning business model in this first inning of AI may not look like your traditional SaaS approach.
So, let’s dive in.
We agree with many great AI experts that in just a couple of years, we will see much more consolidation around roughly two major paradigms for AI, including:
1. A handful of dominant big tech companies that provide generic LLM capabilities.
Foundational LLMs like GPT-4 and Bard, as well as their open-source counterparts in Meta’s LLaMA or Google’s PaLM 2, will continue to evolve and refine their architecture and capabilities.
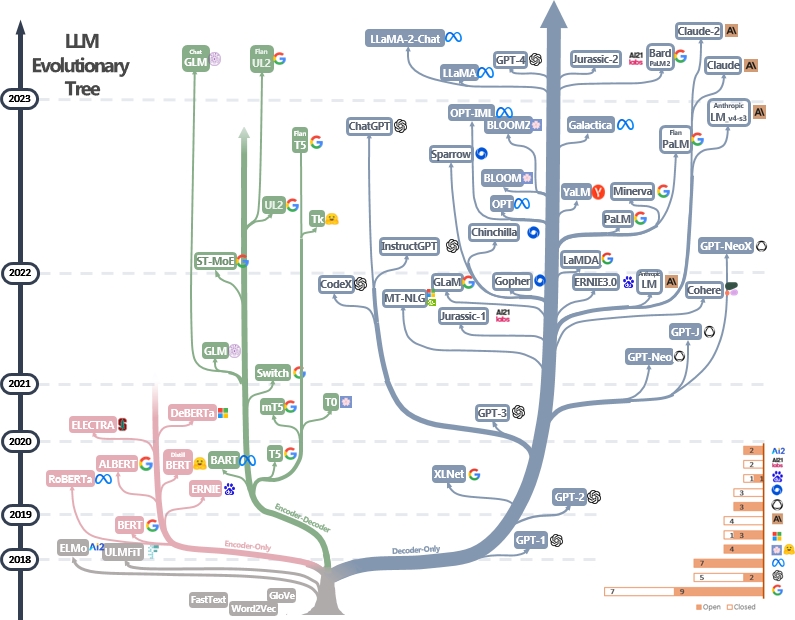
We currently see an initial expansion and branching of the models, but eventually network effects will take hold, and massive consolidation will mirror that of what we saw in the mobile OS world, with Android and iOS eventually dominating the majority of the market share for mobile operating systems.
This consolidation will happen relatively quickly, especially considering the high operational costs of maintaining the infrastructure for its hardware, software, data management, inference capabilities, and security needs. While the total number of dominant generic LLMs will likely number more than just two, there won’t be more than a handful at most.
Nevertheless, these highly intelligent but broadly applicable LLMs will be available to anyone, so no startup can claim a long-term advantage just by providing a mere UI that runs on top of these models. While it can prove valuable in the short term, given the unprecedented access to LLMs and the market’s rush to capitalize on them, it is unlikely to result in a lasting competitive advantage.
This reminds us of the early days of search engines, when a number of startups in the lead gen space were funded by pitching a long-term edge in their ability to arbitrage between Google’s SEO and SEM (organic vs. paid traffic); that window of opportunity closed quickly, and so that strategy eventually failed. Ultimately, the startups that thrived were the ones that created something wholly differentiated, such as ecommerce companies who built additional value on top of the new tech with a logistics infrastructure and strong existing brand.
Similarly, today’s AI startups must have something proprietary and difficult to replicate in order to thrive in the long term. Focusing on fine-tuned AI models combined with the necessary proprietary data could be the answer we are looking for.
2. Fine-tuned models focused on specific sectors and functions.
This narrower focus will give startups the best opportunity to challenge incumbents and defend themselves from scrappy new entrants (e.g., the next YC batch). Models that are trained on specific data sets, enriched with a combination of public and private data, and are solving for a particular verticalized sector or function — such as a fully-automated, lightning-fast multilingual customer service line, a virtual assistant to draft briefs and analyze case law for a legal firm, or a clinician’s assistant to provide diagnosis assistance and drug interaction analysis — will be the most valuable in this first inning of LLM application layer solutions.
This is where startups could build something special, but some key questions remain: What is it about the fine–tuned models that make them so differentiated? For startups that choose to go down this path, what customers should they initially target, and which business models are best suited to solve those customers’ problems?
First, we should consider the ingredients that fine-tuned LLMs need in order to succeed beyond the initial hype.
If we think of an AI “brain” as simplistically as a human brain in its earliest stage of growth, we know that there are two things it depends on for proper development: processing power and fuel.
Processing power, whether it’s hardware in the form of GPUs or software in the form of algorithms, is not in itself a differentiator. The specificity of fine-tuned LLMs means that they are far more operationally efficient than their generic counterparts, which have to be ready to address any possible query. In other words, startups that claim to have a better algorithm may have a short-term advantage, but it won’t be enough to build a business that will win the race to relevance. Thus, similar to the human brain, what we really need in order to grow capacity and quality of output is fuel.
The holy grail lies with what feeds the AI model — relevant, proprietary data sets.
What keys an LLM to serve a particular vertical is proprietary (or unique) data: relevant, domain-specific, and contextual information from a blend of public and private sources. And, as with most training, the more repetition that is involved, the more drastic results you’ll see.
Proprietary and relevant data sets can create a compelling network effect where the machine learning model improves its output quality and increases customer value the more data it gets fed.
This creates a flywheel where fine-tuning a model and providing it with high-quality proprietary data = more value for clients = higher usage = more data that is generated to improve the model = more opportunity to build better products.
.png)
One glaring issue: proprietary data is the name of the game, but startups, by definition, do not have it, while incumbents such as Meta and Salesforce already have more than they know what to do with.
Although startups should be aware of this data disadvantage, it is not as intimidating as it may first appear; even Goliaths require additional specialized engineering or model architecture to fully unlock their potential. In many cases, a custom LLM with the right intuition and context can yield better, faster results than a more generic model with access to a broader data set. Nevertheless, founders should still operate under the assumption that incumbents will have the resources available to craft those custom LLMs, so how are startups meant to compete? How does David beat Goliath?
The Proprietary Data Access Framework
We’ll start with a simple framework that we’ve used to evaluate countless companies to explain how a startup could quickly acquire and utilize their client’s proprietary data to train the fine-tuned, vertical-specific models they need in order to build that moat quickly.
One dimension is private vs. public data, and the other is unstructured vs. structured data. Structured data is highly organized and formatted so that it is easily searchable in relational databases, while unstructured data has no predefined format or organization, making it much more difficult to collect, process, and analyze. However, generative AI has been particularly revolutionary when it comes to analyzing and learning from unstructured data, so we would argue that for the first time in computing history, we now have a more accessible way to analyze visual data in all four quadrants.
.png)
In the first quadrant, we see unstructured private/internal data. Many companies are sitting on large amounts of unstructured data. Examples include recent customer feedback, voice transcripts, emails, videos, surveys, NPS data, and internal manuals for HR, product, and sales teams.
In the second, we have structured private/internal data. Examples of these include historical churn data, financials, quantitative HR records, or CRM data.
In the third, we see unstructured public/external data. This type of data is public and available to anybody, but is still considered differentiated when combined with private data due to its contextual relevance, which allows the model to make sense of it from the perspective of the sector or function it is trying to address. Examples include social posts, articles, or public podcasts or videos on a relevant topic.
And lastly, in the fourth quadrant, we have structured public/external data. Examples include public company financial filings or market research. There are also many federal, state, and local government databases such as the Department of Education’s Open Data Platform or the U.S. Bureau of Labor Statistics's multitude of databases. Contextually relevant competitors’ data, if available, are also very valuable for the model.
The real challenge for training a fine-tuned model is leveraging both private and highly relevant public data. If the public data is not contextualized using the private data, then its value diminishes.
If building the right model architecture and combining it with proprietary data is the name of the game, who you play that game with becomes critical. Choose your customers wisely.
In order for startups to take full advantage of this private data from their customers, they will need to co-mingle the data across all available clients; this blend is what allows the machine to learn effectively. Startups should avoid siloing data off from each other, and instead work to get the proper data permissions from clients. This may be very tricky, even if the data is completely safe and anonymized.
Data partnerships are a great way to build a moat quickly and effectively. So, as the startup is building their go-to-market motion, they may want to prioritize clients who would be more willing to give these permissions in exchange for product value. Highly data-sensitive or heavily-regulated industries, like banking or insurance, for example, would not be the best place to search for first adopters.
Not surprisingly, large public enterprises have more stringent requirements for accessing their data; they will likely not want their data to be co-mingled across the startup’s other clients. On the other hand, SMBs in industries with less sensitivity to data sharing may be more willing, as long as the value exchange is positive for them as well. Clients who have an urgent need to implement AI may also be more willing to share data; this urgency may come from competitive pressures or clear ROI improvements. At this stage, the startup should be ruthless in prioritizing customers who give them these permissions.
.png)
Data access and utilization will also heavily depend on the business models startups choose to pursue.
One very important additional consideration is what type of business model will give founders the proprietary data needed to build great products.
On one end, we have SaaS models that sell the software to a client, who then inject their own workflows and data for a more “self-serve" approach. While this approach could work for the first inning of AI-enabled products, perhaps the better model would be a managed service model, in which the client asks the startup to run data through their model. The company would then provide their client with only the insights or answers that were asked of them. This would be more akin to a “do it for me” model.
We have a rich history of managed service business models profiting off of open-source movements. In the 1990s and 2000s, startups that initially acted as managed service providers for powerful new open-source tech ended up dominating a large portion of the market. Namely, Red Hat Software supported Linux, Cloudera supported Hadoop, and GitHub supported Git. We see a similar set of fine-tuned sector- or function-specific winners set to emerge from this AI wave.
A managed service business model works for clients who do not have the proper in-house AI expertise, and as a result is especially advantageous for startups who can hire AI talent to solve problems for a multitude of less-technically-savvy clients. Gartner’s recent analysis around the shortage of great AI engineering talent bodes well for startups, who are better-positioned to lure great talent seeking a nimble and cutting-edge working environment.
.png)
In summary, we are seeing a handful of best practices emerging that can help founders nail down the critical dimensions of a successful and defensible AI startup:
- Build differentiated value by adapting the model for its intended purpose with the proprietary data needed to solve the customer problem. While many companies build on top of LLMs, many are not building differentiated value or defensibility against incumbents. Proprietary data is the fuel that enables companies to build unique, lasting products over time, and make it harder for competitors to replicate them.
- Consider the 4 ways to think about proprietary data access; what you can access will determine the products and features you can build. Founders who make it a priority to get access to this data through a combination of public, private, structured, and unstructured client sources will differentiate and build more substantial and defensible products.
- Choose your customers and business model wisely. Enterprise, mid-market, and SMBs all have different data sharing sensitivities. Startups must choose the one that best enables them to get the data needed to further refine their LLMs. Consider whether a managed service model works better to access that data than the traditional workflow SaaS business models; does the client want “do-it-yourself” or “do-it-for-me” AI?
The fight against incumbents with more proprietary data and engineering resources will be tough, but it can be won, and the payoff for doing so will be substantial. The key to success will look different for startups than it will for larger companies, allowing founders to redefine the rules of engagement.
David knew he couldn’t win against Goliath in close-range combat, so he refused to play his game. Instead, he defeated the giant from a distance — turning the battlefield on its head by leveraging his own unique agility and ingenuity.
About the author

Roble Ventures is a future-of-work focused fund investing in technologies that enable people, teams, and organizations to achieve their most ambitious work.